Transform Risk into Opportunity
The insurance industry thrives on managing uncertainty, but traditional actuarial models—often rooted in statistical averages and historical data—struggle to keep pace with real-time volatility. Data science shifts this paradigm by integrating dynamic, high-dimensional datasets and predictive algorithms. At its core, Qubitstats leverages machine learning (ML), artificial intelligence (AI), and big data analytics to extract actionable insights from structured (e.g., claims records) and unstructured (e.g., IoT sensor feeds) sources.
For example, instead of relying solely on static demographic tables, Qubitstats could employ gradient boosting models or neural networks to weigh variables like customer behavior, environmental factors, and macroeconomic trends in real time. This fusion of domain expertise and advanced analytics allows insurers to move from reactive risk assessment to proactive opportunity creation—optimizing pricing, reducing exposure, and enhancing customer lifetime value (CLV).
Why Partner with Us?
Our solutions are designed to turn risk management into a competitive edge, empowering insurers to innovate faster and adapt smarter.
From a data science perspective, this is about optimization and differentiation. Qubitstats’ value proposition hinges on its ability to transform raw data into a decision-making engine. This involves not just predictive modeling but also prescriptive analytics—telling insurers what to do based on what will happen. By deploying scalable cloud infrastructure and automated ML pipelines, Qubitstats enables insurers to iterate rapidly, test hypotheses (e.g., A/B testing pricing models), and adapt to market shifts faster than competitors reliant on manual processes or outdated systems.
We turn risk management into your strategic advantage. Our data-driven solutions—powered by automated ML pipelines and prescriptive analytics—enable insurers to innovate at speed, adapt with precision, and outpace the competition.
1. Price Smarter with Dynamic Models
Dynamic pricing is a quintessential application of data science. For telematics, Qubitstats likely uses time-series analysis and clustering algorithms (e.g., K-means) to segment drivers based on granular behavior metrics—acceleration, cornering, nighttime driving—then feeds these into a pricing model (e.g., generalized linear models or random forests) to predict claim likelihood. The 25% payout reduction suggests a strong lift in model accuracy, possibly measured via metrics like Area Under the Curve (AUC) or precision-recall tradeoffs.
For climate risk, Qubitstats could integrate geospatial data (via GIS systems) with ensemble models (e.g., XGBoost) to forecast property-level risk scores. Features like historical weather patterns, soil moisture levels from satellites, and proximity to firebreaks enhance predictive power. This isn’t just about pricing—it’s about portfolio optimization, ensuring premiums reflect true risk exposure.
- Telematics Integration: “Our AI harnesses time-series telematics data—speed, braking, and more—to build precise risk profiles, enabling usage-based pricing that rewards safe drivers.”
- Climate Risk Modeling: “Using geospatial analytics and ensemble models, we fuse satellite imagery with climate trends to dynamically price property risks like wildfires or floods.”
- Example: “A regional auto insurer slashed claim payouts by 25% and grew its low-risk customer base, thanks to our ML-powered usage-based pricing.”
Visualizing driver segmentation with telematics data—Qubitstats’ ML clusters reward safe driving with precise pricing.
2. Prevent Losses with Predictive Analytics
Fraud detection relies on anomaly detection techniques—think isolation forests or autoencoders—trained on claims data to flag outliers (e.g., duplicate invoices, mismatched timelines). Qubitstats likely uses feature engineering to enrich datasets, such as linking claims to external sources (e.g., social media, public records) for corroboration. The $2M fraud ring takedown suggests a high true-positive rate, validated through confusion matrix analysis.
For risk mitigation, supervised learning models predict loss probability, leveraging features like historical claims, IoT signals, or even psychographic data. These insights feed into decision rules—e.g., adjusting deductibles for high-risk flood-zone homes—reducing portfolio tail risk.
- Fraud Detection: “Our ML anomaly detection scans claims for red flags—like inconsistent narratives or duplicates—stopping fraud in its tracks.”
- Risk Mitigation: “Predictive models pinpoint high-risk profiles using IoT and historical data, enabling proactive coverage adjustments.”
- Example: “A health insurer saved $2M by dismantling a prescription fraud ring with our real-time anomaly detection tools.”
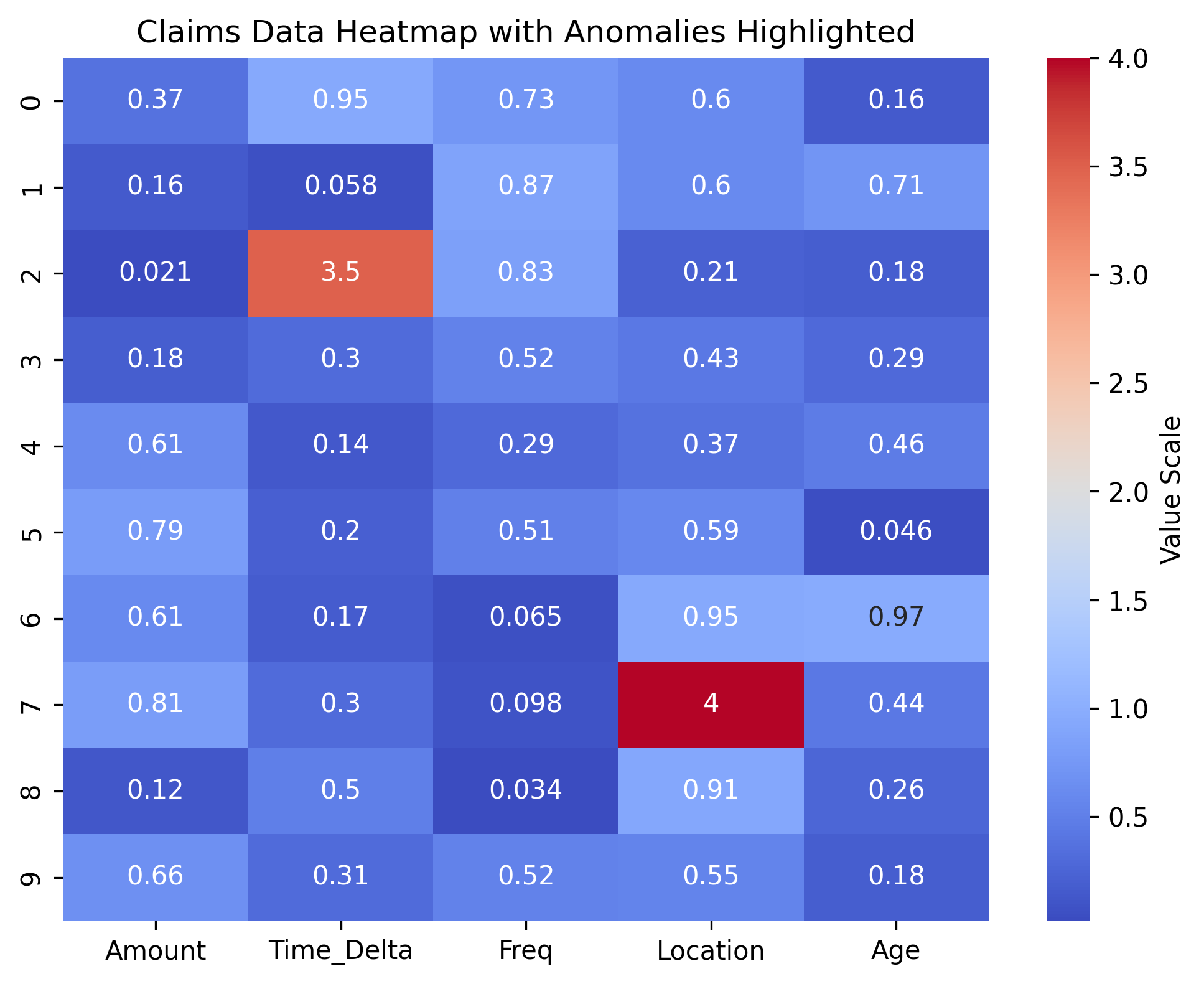
3. Personalize Offerings for Deeper Loyalty
Personalization is powered by unsupervised learning (e.g., clustering customers by behavior) and reinforcement learning (optimizing offers in real time). IoT data from fitness trackers or smart thermostats feeds into feature stores, enabling real-time scoring of risk or reward eligibility. The 18% retention lift likely ties to a churn prediction model—e.g., logistic regression or survival analysis—identifying at-risk customers for targeted interventions like dynamic riders.
Micro-insurance showcases AI’s agility: natural language processing (NLP) could parse customer needs from inquiries, while decision trees assemble modular policies on the fly. This reduces customer acquisition costs (CAC) and boosts CLV.
- Behavioral Analytics: “We tap IoT streams—fitness trackers, smart sensors—to craft personalized discounts for healthy or safe living.”
- Micro-Insurance: “AI assembles modular, on-demand coverage for gig workers or travelers, tailored in real time.”
- Example: “A life insurer lifted retention 18% by deploying dynamic riders, powered by our churn prediction models.”
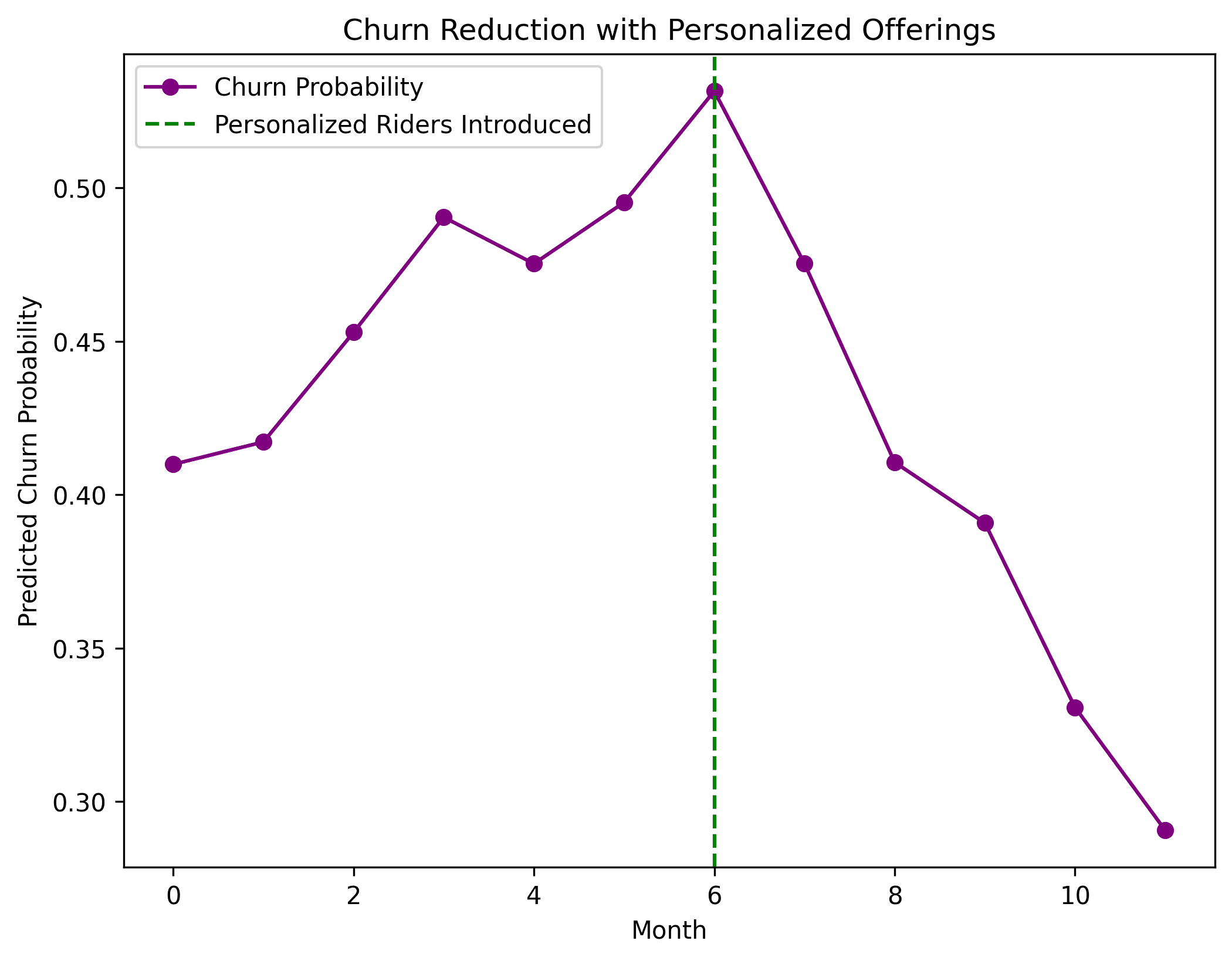
4. Streamline Operations with Intelligent Automation
AI claims triage likely uses convolutional neural networks (CNNs) trained on labeled images (e.g., car dents, roof damage) to classify severity and estimate repair costs, cutting human review time. Chatbots, powered by NLP and intent recognition, handle structured tasks via pre-trained models like BERT, offloading routine inquiries. The 14-day-to-48-hour drop reflects a streamlined pipeline—possibly orchestrated via robotic process automation (RPA) and validated with process mining to eliminate bottlenecks.
- AI Claims Triage: “Our deep learning assesses damage photos instantly, accelerating claims from assessment to payout.”
- Chatbot Assistants: “NLP-driven virtual agents resolve inquiries 24/7, slashing call center loads by up to 40%.”
- Example: “A global P&C insurer cut claims processing from 14 days to 48 hours with our end-to-end automation suite.”
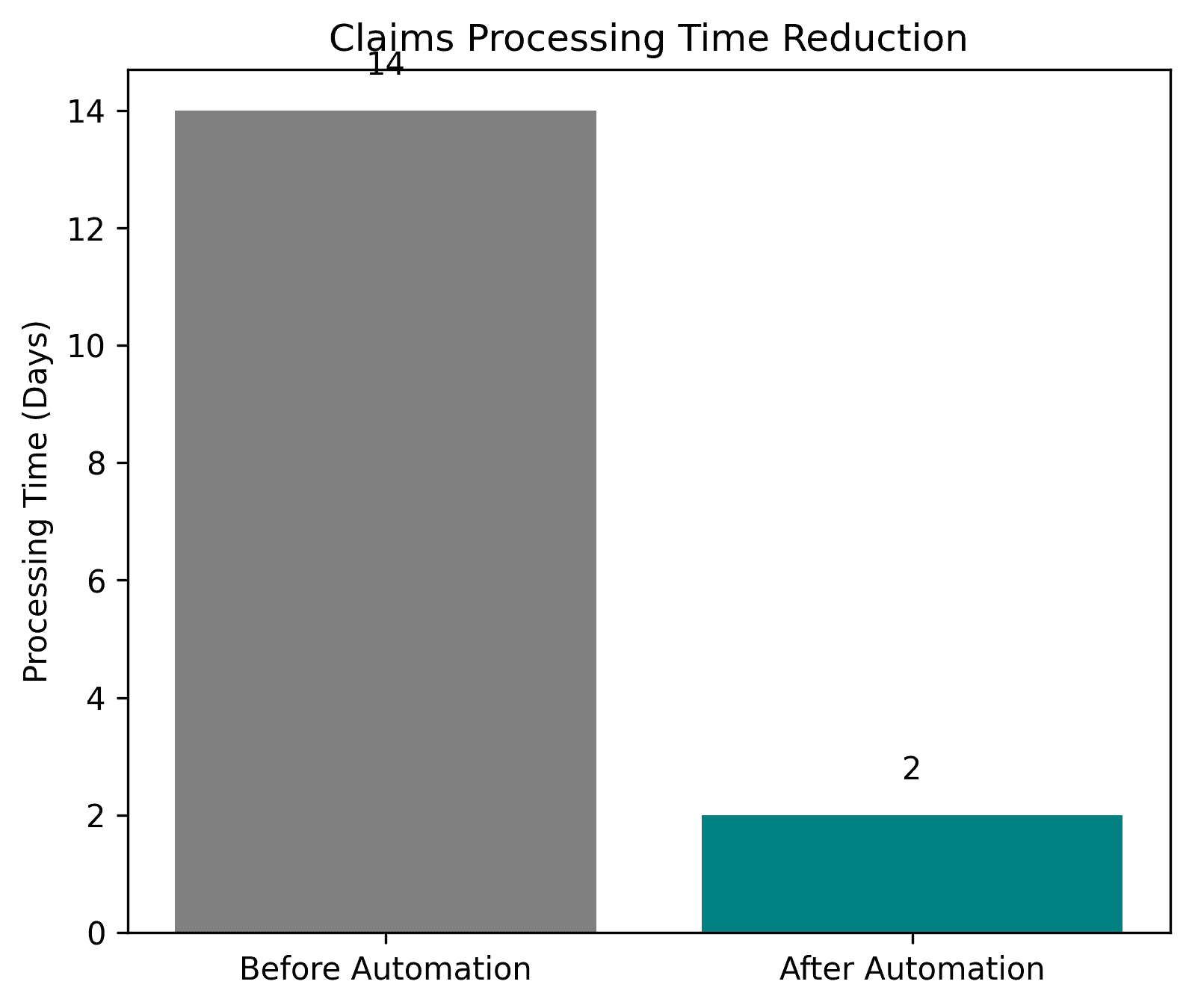
Tailored Solutions for Every Sector
Life & Health, Property & Casualty, Reinsurance examples.
Each sector benefits from bespoke analytics: survival models for life underwriting, Monte Carlo simulations for catastrophe modeling, or portfolio optimization via stochastic gradient descent for reinsurance. These aren’t just tools—they’re frameworks for translating domain-specific data into measurable outcomes.
From survival analysis in life insurance to Monte Carlo risk simulations for P&C, our sector-specific analytics deliver precision and impact.
Case Study Spotlight
- Challenge: Rising weather-related losses.
- Solution: “We engineered a geospatial platform blending satellite data, climate forecasts, and structural insights into a predictive risk engine.”
- Result: “High-risk renewals dropped 30%, and weather-related claims fell 15% in 18 months.”
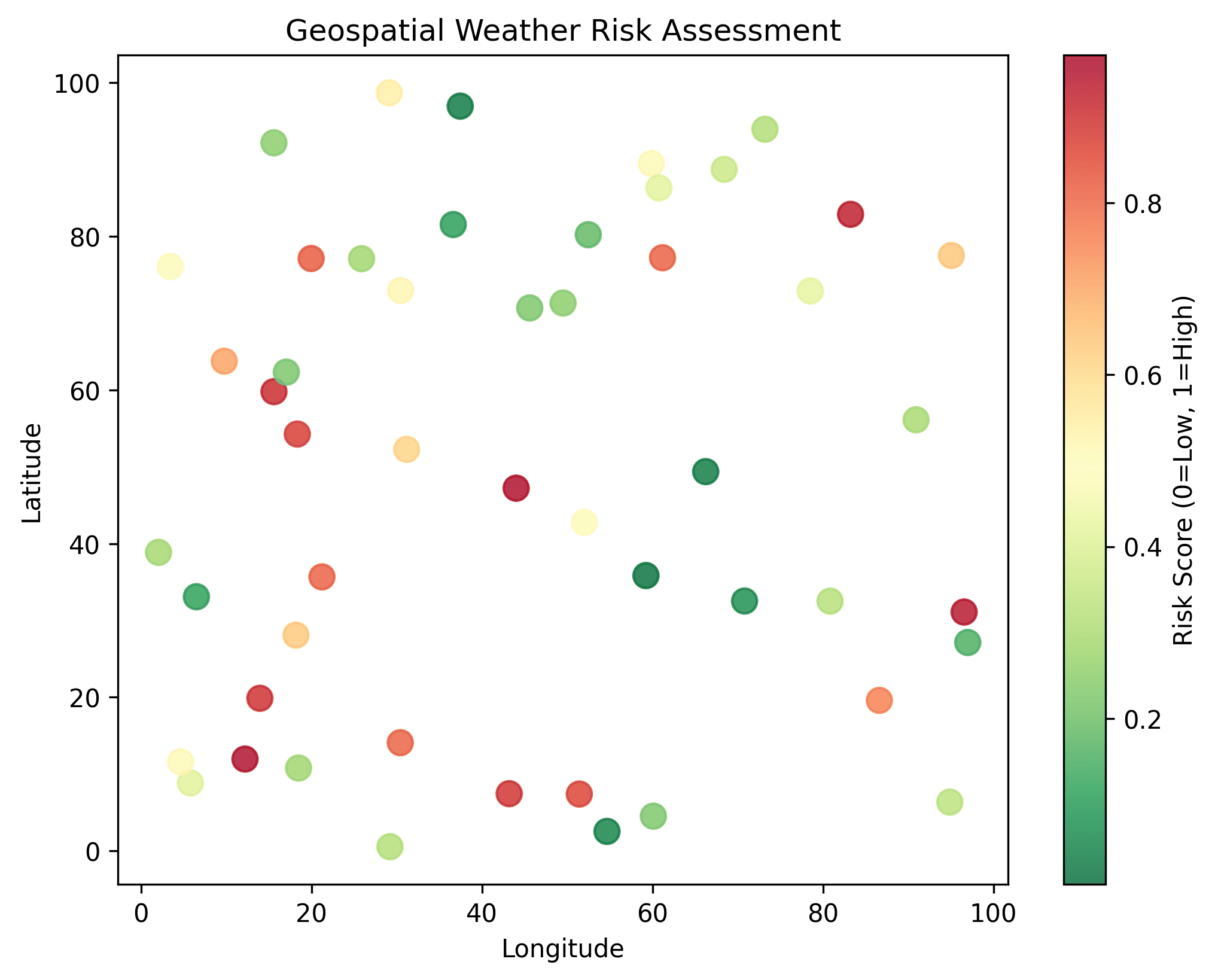
The geospatial platform likely integrates raster data (satellite imagery) with vector data (building codes) into a feature-rich dataset, processed via a random forest or gradient boosting model to classify risk. The 30% renewal reduction suggests a binary classification model with high specificity, while the 15% claims drop reflects improved exposure management—quantifiable via loss ratio analysis.
Lead the Future of Insurance
The future is about real-time data ecosystems—edge computing for IoT, federated learning for privacy-preserving personalization, and API-driven embedded insurance. Qubitstats’ strength lies in orchestrating these technologies into a cohesive strategy.
From IoT-powered policies to insurance embedded in smart ecosystems, we equip you to lead with agility and insight.